Review It refers to any stimulus that can interfere with or degrade the quality of a message.
Mẹo Hướng dẫn It refers to any stimulus that can interfere with or degrade the quality of a message. Mới Nhất
Lê Minh Phương đang tìm kiếm từ khóa It refers to any stimulus that can interfere with or degrade the quality of a message. được Cập Nhật vào lúc : 2022-11-10 06:22:02 . Với phương châm chia sẻ Bí quyết về trong nội dung bài viết một cách Chi Tiết Mới Nhất. Nếu sau khi tham khảo tài liệu vẫn ko hiểu thì hoàn toàn có thể lại Comments ở cuối bài để Admin lý giải và hướng dẫn lại nha.INTRODUCTION
Everyday listening frequently occurs in the context of acoustic challenges that degrade the auditory signal (Mattys et al. 2012). External sources of acoustic challenge include background noise, competing speech, or talkers with a foreign accent (Van Engen & Peelle 2014). Even when the external auditory signal is perfectly clear, hearing impairment reduces the fidelity of the information reaching a listener’s perceptual system. External and internal sources of interference therefore combine to challenge listeners’ comprehension an acoustic level. How do we make sense of acoustically degraded speech, particularly with the speed and efficiency required during everyday conversation?
Nội dung chính Show- INTRODUCTIONUNDERSTANDING ACOUSTICALLY DEGRADED SPEECH REQUIRES COGNITIVE
PROCESSINGBehavioral Evidence for Cognitive Challenge During ListeningPhysiological Evidence for Cognitive Challenge During ListeningNeuroimaging Evidence for Cognitive Challenge During ListeningCognitive Processing During Listening: A SummaryCOGNITIVE PROCESSES IMPLICATED IN COMPREHENDING DEGRADED SPEECHVerbal Working MemoryAttention-Based Performance MonitoringFlexible Allocation of Neurocognitive ResourcesThe Role of Cognitive Factors in Explaining Individual Differences in Speech UnderstandingListening Effort and Neural PlasticityCONCLUSIONSACKNOWLEDGMENTSWhich term refers to the means by which such a message is conveyed?What is the term for forces that can impede or obstruct a communication interaction and can be internal or external?What are the standards of right and wrong one applies to messages that are sent and received?What term describes receiving and interpreting messages from others?
Below I review evidence from a variety of sources supporting the basic claim that understanding acoustically degraded speech requires that listeners engage cognitive resources, as well as evidence for the specific processes involved. Evidence from multiple experimental approaches demonstrates that cognitive aspects of listening effort are real, measurable, and informative with respect to both theoretical and practical aspects of speech understanding. Various types of acoustic challenge affect different aspects of the speech signal. For example, in noise vocoded speech (Shannon et al. 1995) information is “accurate” but low in spectral detail, whereas in the presence of background noise some acoustic information is masked. It is likely that the specific type of acoustic degradation influences the cognitive processes listeners use. However, investigating these differences is beyond the scope of the current review.
UNDERSTANDING ACOUSTICALLY DEGRADED SPEECH REQUIRES COGNITIVE PROCESSING
When listeners hear speech they must match the rapid incoming acoustic stream to stored representations of words and phonemes to successfully extract the intended meaning. The process of correctly identifying sounds is made more difficult when speech is acoustically degraded: less information is available to the listener, which reduces the quality of speech cues and thus increases the chance for error. As illustrated in Figure 1A, the acoustic challenge associated with any stimulus depends on the abilities of a particular listener, the clarity of the external signal, and the acoustic environment (see the classic “speech chain” of Denes & Pinson 1993, as well as the more recent Mattys et al. 2012). In the following sections, I will only be able to consider a subset of these conditions, but it is easy to imagine how different types of acoustic degradation might challenge listeners’ auditory and cognitive systems.
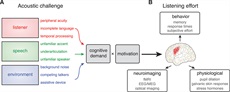
A, The overall acoustic challenge experienced by a given listener is a combination of individual hearing ability and external acoustic characteristics (including speech quality and background noise). (Note only a subset of these conditions are directly addressed in the main text.) Acoustic challenge increases cognitive demand, which is a key contributor to listening effort (moderated by motivation). When speech is not easily matched to a listener’s expectation, additional neural processing is frequently required. B, Increases in listening effort can be observed through functional brain imaging, are reflected in physiological responses outside the brain, and frequently result in measurable differences in behavior.
At the outset, it is useful to draw a distinction between listening demand and listening effort. Listening demand reflects the various challenges associated with a given listening situation, including challenges to the acoustic signal (as illustrated in Fig. 1A) and other demands of a given situation (such as language processing). Instead of listening demand, I will use the term cognitive demand to emphasize cognitive processes involved in understanding acoustically degraded speech. As illustrated in Figure 1, increased acoustic challenge results in greater cognitive demand, which—modulated by a listener’s motivation—leads to increased listening effort. Here, my focus is on increased cognitive processing associated with effortful listening: that is, listeners are forced to rely to a greater extent on cognitive systems to successfully extract meaning from an acoustically degraded speech signal compared with a clear signal.
In contrast to cognitive demand, listening effort refers to the resources or energy actually used by a listener to meet cognitive demands. A recent consensus paper proposed the Framework for Understanding Effortful Listening, comprehensively addressing many of the complexities that go into concepts of spoken communication and listening effort (Pichora-Fuller et al. 2022 and the accompanying special issue). Pichora-Fuller et al. (2022) define listening effort as “the deliberate allocation of mental resources to overcome obstacles in goal pursuit when carrying out a task, with listening effort applying more specifically when tasks involve listening” (Pichora-Fuller et al. 2022, page 5S), which emphasizes the distinction between the demand of a given listening situation and the effort a particular listener exerts. In the sections below, rather than attempt to cover the entire spectrum of effortful listening, I make the case that acoustic challenge leads to cognitive demand and provide a framework for thinking about what specific cognitive processes might be involved in understanding acoustically degraded speech. However, it is important to keep in mind that there are other factors that relate to listening effort, including (but not limited to) motivation (Eckert et al. 2022; Richter 2022), fatigue (Hornsby et al. 2022), and psychosocial considerations (Pichora-Fuller 2022). I return to the important issue of motivation below, but throughout the following discussion my assumption is that listeners are motivated to understand what they are hearing. That is, I will refer to a straightforward relationship between acoustic challenge and listening effort to focus on the cognitive processes engaged. As illustrated in Figure 1B, the cognitive response to acoustic challenge is evident in neuroimaging measures of brain activity and reflected in physiological responses and behavior. Converging evidence that cognitive resources are required to understand degraded speech comes from each of these sources.
One important question is whether the cognitive resources required to understand acoustically degraded speech are specific to auditory processing or whether they involve mechanisms that operate across a variety of tasks. The latter processes are often referred to as “domain general” because they involve operations that might need to occur similarly regardless of the input modality of a stimulus. Domain-general processes are often associated with executive tasks such as decision making, error detection, and task switching (Baddeley 1986,1996). The neural support for domain-general processes is typically associated with frontal and parietal cortex, regions that have strong anatomical connectivity to primary sensory cortices and which contain neurons that change their activity to reflect current task demands (Antzoulatos & Miller 2022; Stokes et al. 2013). Throughout the sections below, a recurring theme will be considering whether the results are consistent with a role for domain-general systems in listening effort.
Behavioral Evidence for Cognitive Challenge During Listening
Hearing impairment and other forms of acoustic challenge have long been associated with increased difficulty processing and remembering speech across a wide range of tasks and stimuli. When hearing speech that is acoustically degraded, listeners are not only less accurate perception, but take longer to produce responses (Gatehouse & Gordon 1990). Even when speech is understood, words or syllables that are acoustically degraded are more difficult to remember (Heinrich et al. 2008; Surprenant 1999), an effect exacerbated in older adults (Heinrich & Schneider 2011; Murphy et al. 2000; Pichora-Fuller et al. 1995). Sentence processing is also affected, such that listeners with poorer hearing make more errors when processing syntactically complex sentences (DeCaro et al. 2022; Wingfield et al. 2006).
When degraded speech itself is remembered less well, there is a possibility that a listener’s difficulty occurs the level of perception, despite apparently preserved audibility. That is, if a word is not correctly heard in the first place, it cannot be remembered. In an elegant study examining the influence of acoustic challenge on episodic memory, Rabbitt (1968) addressed this possibility. Participants were presented with lists of spoken digits: the first portion of each list contained unprocessed speech, with the second portion presented in noise. Critically, memory for the early list items—which themselves were not degraded—was poorer when items in the later part of the list were degraded, suggesting that understanding speech in noise interfered with cognitive processes required for memory encoding. This effect has been replicated in word lists (Cousins et al. 2014; Piquado, Cousins, et al. 2010) and is also present in running memory for speech (McCoy et al. 2005). These studies present compelling evidence that acoustic challenge affects nonacoustic tasks (in this case, memory for what has been correctly heard), pointing toward the involvement of domain-general cognitive resources in understanding degraded speech.
Although many memory studies have used single words, acoustic challenge can also reduce comprehension and memory in the context of short stories (Piquado et al. 2012; Rabbitt 1991; Ward et al. 2022). Piquado et al. (2012) found that hearing-impaired listeners showed poorer memory for short stories compared with listeners with normal hearing, and further that memory difficulties were reduced when listeners were allowed to slow the rate of story presentation through self-paced listening. The fact that increased processing time improved performance for listeners with hearing impairment more than for listeners with normal hearing suggests that hearing-impaired listeners were under a greater degree of cognitive challenge (Wingfield et al. 1999).
Another approach to studying the role of cognitive factors in speech understanding comes from using visual tests (which avoid acoustic processing challenges) to assess cognitive ability. The rationale is that domain-general, shared cognitive resources (such as attention, verbal working memory, and ability to use semantic context) will operate on linguistic material regardless of the input modality. Zekveld et al. (2007) developed the Text Reception Threshold (TRT) test, a visual analog of the standard Speech Reception Threshold (SRT) test, using visually degraded written sentences as stimuli. TRT thresholds significantly correlate with SRT, even when controlling for age (Besser et al. 2012; Kramer et al. 2009). Because the TRT does not involve auditory processing, the correlation between TRT and SRT scores is consistent with an important role for extra-auditory cognitive processes in speech understanding.
Thus, there is good evidence across a variety of behavioral tasks that auditory processing relies on domain-general cognitive resources to a greater degree when speech input is acoustically degraded.
Physiological Evidence for Cognitive Challenge During Listening
Pupil diameter increases as a function of momentary cognitive demand (Kahneman & Beatty 1966) and thus provides an online measure of cognitive effort independent of a behavioral task (Laeng et al. 2012). Pupil dilation is generally attributed to activity in the locus coeruleus (Aston-Jones & Cohen 2005; Koss 1986) and can thus be considered an indirect measure of neural activity accompanying cognitively demanding listening: The expectation would be that when speech processing is cognitively demanding, pupil dilation would be observed [Common measures of pupil response include dilation amplitude, peak latency, and mean pupil dilation, which may reflect different physiological responses. Variants of linear mixed effects analysis using flexible basis sets have also seen increasing use in analysis of pupillometric data (Mirman 2014). It is also important to note that factors other than task difficulty (e.g., reward or valence) can influence the pupil response, so changes in pupil dilation are not necessarily indicative of cognitive processing.]. An advantage of pupillometry is that it provides an indication of changes in cognitive demand during perception (an “online” measure) rather than measuring changes that occur after perception has occurred, such as word repetition (an “offline” measure). Because pupil dilation is a continuous measure, it is also possible to dynamically track it as it unfolds over time, rather than being restricted to a single response (e.g., word repetition).
Indeed, pupil diameter increases with acoustic challenge across a variety of listening situations. In young adults, increased pupil dilation is seen during sentence processing as a function of signal-to-noise ratio (SNR), with these effects amplified in listeners with poor hearing more positive SNRs (Kramer et al. 1997; Zekveld & Kramer 2014; Zekveld et al. 2010). In older adults, pupil responses are altered due to restricted dynamic range, eyelid position (i.e., droop), and corneal refraction; nevertheless, it is possible to correct for these issues and obtain good quality data (Piquado, Isaacowitz, et al. 2010). In older adults, we might expect to see increases in cognitive challenge due to a combination of change in both cognitive and auditory ability. In practice, however, older adults have been shown to be less responsive to changes in SNR than are young adults (Zekveld et al. 2011). One explanation for these results is that for any condition involving speech understanding in noise, older adults are already using additional cognitive resources (Wingfield et al. 2005). Thus, the possible range of older adults’ pupil response to task manipulations is reduced, as they may already be recruiting additional cognitive resources in the easiest condition.
Pupil dilation reflects cognitive processing that can arise not only from acoustic degradation but also from linguistic challenge. For example, in the context of lexical competition, words with a large number of competing targets (e.g., “cat” might be confused for “cap,” “cat,” etc.) are more difficult to accurately perceive (Luce & Pisoni 1998; Marslen-Wilson & Tyler 1980). Behaviorally, older adults are differentially affected by lexical competition (Sommers 1996); pupillometry confirms that older adults with hearing loss are impacted by SNR, lexical competition, and the interaction of the two sources of difficulty (Kuchinsky et al. 2013). Thus, pupillometry captures challenges related to both acoustic and linguistic factors.
Neuroimaging Evidence for Cognitive Challenge During Listening
Behavioral and physiological measures are useful because they reflect changes in neural processing associated with increased acoustic challenge; functional neuroimaging permits the measurement of neural activity in a more direct fashion (Evans & McGettigan 2022). Although care must be taken when using functional magnetic resonance imaging to study auditory function due to the acoustic noise generated by the scanner, it is possible to obtain data regarding neural responses to speech (Peelle 2014). Other methods are able to provide localized measures of brain activity in quiet, including EEG and magnetoencephalography (Wöstmann et al. 2022) and optical neuroimaging (Peelle 2022).
The brain networks involved in speech understanding have most frequently been studied by looking for regions that show increased activity for intelligible speech compared with an unintelligible control condition, such as noise-vocoded or spectrally rotated speech (Scott et al. 2000). Across different laboratories and stimuli, functional neuroimaging studies consistently find intelligible sentences are processed by bilateral temporal cortex, frequently complemented by inferior frontal gyrus (Crinion et al. 2003; Davis et al. 2011; Davis & Johnsrude 2003; Evans et al. 2022; Hassanpour et al. 2015; McGettigan et al. 2012; Obleser et al. 2007; Okada et al. 2010; Peelle, Eason, et al. 2010; Rodd et al. 2005; Rodd et al. 2010). These regions form a functional hierarchy, with regions nearer to auditory cortex showing increased response to acoustic features, and regions further removed a greater degree of acoustic invariance (that is, responding similarly regardless of how the speech was degraded) (Davis & Johnsrude 2003).
To identify neural signatures of listening effort, it is also helpful to consider the converse—that is, regions that show an increased response for degraded (yet still intelligible) speech. In practice, this has often involved comparing brain activity when listening to speech with less-than-perfect intelligibility (e.g., noise-vocoded speech with four or six channels) to that for intelligible speech (e.g., unprocessed speech). Using a correlational approach with sentences that parametrically varied in intelligibility, Davis and Johnsrude (2003) found increased neural activation for degraded speech in left lateral temporal cortex, inferior frontal cortex, and premotor cortex. These regions displayed an inverse U-shaped function, showing activity for slightly degraded speech that was larger than for unprocessed speech and also greater than that seen for severely degraded speech (consistent with an effort-related response). Of particular importance is the fact that the stimuli included several different types of acoustic degradation; the degradation-related increases in activity in temporal cortex differed depending on the acoustic characteristics, but the degradation-related increases in frontal cortex did not. The acoustic invariance of the response in frontal cortex is consistent with the hypothesis that similar executive processes, supported by regions of frontal cortex, are involved in extracting meaning from a degraded speech signal regardless of the specific acoustic features of that signal (Davis & Johnsrude 2007; Peelle, Johnsrude, et al. 2010).
Increased activity for degraded speech is also frequently observed in the cingulo-opercular network (anterior cingulate and bilateral anterior insulae) during conditions in which intelligibility suffers (Eckert et al. 2009; Erb et al. 2013; Vaden et al. 2013; Wild, Yusuf, et al. 2012). The involvement of the cingulo-opercular network when listeners make identification errors is consistent with a response to error-monitoring or attention, a topic that I address in more detail below. At this point, it is simply worth noting that there are several anatomically distinct brain networks that show a differential response to acoustically degraded speech.
It is useful to consider whether effort-related increases are observed in domain-specific auditory or language systems, or domain-general executive systems. Many of the regions showing effort-related increases in activity fall within the multiple-demand system, a constellation of cortical regions that show increased activity across a wide variety of tasks and modalities (Duncan 2010) and which can be broken into multiple attention-related subsystems, including the frontoparietal and cingulo-opercular networks (Neta et al. 2015; Power & Petersen 2013). The anatomical location of effort-related neural activity is therefore broadly consistent with executive processes required to support speech understanding (Wingfield & Grossman 2006).
Thus, although the specific anatomical distribution shows some variability across study, there are several regions of the brain that are consistently more active when listeners process acoustically degraded speech compared with normal speech )(supported by a meta-analysis from Adank 2012).
There are also brain regions that show the opposite pattern: that is, increased responses when speech is acoustically clearer compared with when it is acoustically degraded (Evans et al. 2014; Lee et al. 2022; Wild, Davis, et al. 2012). These may reflect the greater availability of prosodic information, acoustic cues to talker characteristics, or listeners’ ability to more deeply process linguistic information because it is more intelligible.
Cognitive Processing During Listening: A Summary
Converging evidence from diverse experimental approaches points toward increased cognitive processing when listeners process degraded speech. These changes in cognitive processing are evident in functional brain imaging, can lead to changes in pupil dilation, and are ultimately reflected in listeners’ behavior.
Figure 2A shows a schematic of listening effort that emphasizes the importance not only of cognitive demands but a listener’s motivation to understand. That is, if a listener has little motivation to understand what they are hearing, increasing cognitive demands may result in little or no change in effort. Cognitive demand includes acoustic challenge (which has been my primary focus) but is also affected by factors including linguistic challenge, cognitive ability, and language ability. These contributors are critical to understand because of their relationship to cognitive demand, but their relationship to listening effort is mediated by a listener’s motivation.

Cognitive demands during listening. A, Schematic of listening effort as a function of motivation and cognitive demand (after Pichora-Fuller et al. 2022). Although many factors can affect cognitive demand during listening, this cognitive demand is moderated by a listener’s motivation. Note that in addition to acoustic challenge (see Figure 1) there are other factors that influence cognitive demand, including the linguistic complexity of the speech and cognitive and linguistic abilities of individual listeners. B, Listeners typically expend more resources as acoustic clarity decreases until acoustic challenge becomes too difficult, which point effort decreases. Listeners with lower cognitive ability reach this point sooner due to relatively fewer cognitive resources than listeners with higher cognitive ability. C, When acoustic challenge is low, accuracy of speech understanding remains high; as speech is increasingly degraded, perception accuracy drops off despite increased effort. Accuracy drops off more quickly for listeners with lower cognitive ability.
A few key points are worth noting. First, cognitive processing during speech understanding is almost certainly not an all-or-none response but reflects the acoustic (and linguistic) challenge of a given situation: listening effort will typically increase in proportion to demand (depending on motivation). Figures 2B and 2C schematically illustrate a simplified relationship between acoustic challenge and listening effort, assuming a listener is motivated to understand, to make additional points. At low levels of acoustic challenge, speech understanding is largely automatic; comprehension accuracy is generally high in this situation. As acoustic challenge increases, more cognitive processing is needed to understand speech. As shown in Figure 2C, for many conditions of moderate acoustic challenge, behavioral performance remains high—that is, speech can be accurately understood, despite some degradation in the signal, but a cost of additional effort. At more severe levels of acoustic challenge, however, performance may drop off, despite increased effort. This does not necessarily prevent communication, but it may make comprehension more difficult. For example, we may not catch every word of a conversation in a noisy restaurant, but we understand enough to generally follow along and join in. At extremely high levels of acoustic challenge, effort may decrease if listeners determine that they will not be successful comprehension (Eckert et al. 2022; Kukla 1972; Richter 2022). In this case, effort is reduced, but comprehension accuracy is poor because the listener is not able to meet the current cognitive demand. (This can be compared with a situation with acoustically clear, nonchallenging speech in which effort is low, but comprehension is high because the cognitive demands are also low.)
It is also important to consider individual differences in cognitive ability. For example, in the case of verbal working memory, we might measure a listener’s ability using a behavioral memory task and find that some listeners are able to correctly remember more items than other listeners. However, given that multiple cognitive processes are involved in understanding degraded speech, it can be useful to think about cognitive ability in the abstract (Wingfield 2022). As shown in Figure 2B and Figure 2C, listeners with lower cognitive ability will find speech generally more difficult to understand, even low levels of acoustic challenge. As acoustic challenge increases, the lack of available cognitive resources may lead to increased listening effort, or decreased accuracy, compared with listeners with a high cognitive ability. Differences in cognitive ability can be measured the level of a participant group (normal hearing versus hearing-impaired patients, young adults versus older adults), or simply individual variability in cognitive ability assessed using behavioral measures (Grady 2012).
COGNITIVE PROCESSES IMPLICATED IN COMPREHENDING DEGRADED SPEECH
Evidence supporting a role for cognitive resources in understanding acoustically degraded speech is wide-ranging and replicable. However, less is known about the specific cognitive processes engaged. Do listeners rely on a single cognitive network when speech is acoustically challenging or are there dissociable processes that are selectively recruited depending on the situation? Below I review evidence for the involvement of least two cognitive systems in comprehending degraded speech: verbal working memory and attention-based performance monitoring.
Verbal Working Memory
One of the more compelling suggestions in the literature is that acoustically degraded speech requires listeners to rely to a greater extent on verbal working memory (Rabbitt 1968; Rönnberg et al. 2013; Rönnberg et al. 2008; Wingfield et al. 2015). In this context it is valuable to distinguish between two related types of memory. Short-term memory typically refers to the ability to maintain information in mind (for example, remembering a phone number long enough to write it down), whereas working memory involves both the maintenance and manipulation of information (for example, putting the digits of a phone number in ascending order; Baddeley 1986). These two constructs are emphasized to varying degrees in different studies. For simplicity, I will refer to “verbal working memory,” but the contributions of multiple components of verbal memory is a nontrivial distinction and becomes particularly relevant when attempting to identify cognitive tests to understand individual differences in speech understanding performance.
An intuitive way to think about the role of verbal working memory is that if an incoming signal cannot be understood, it must be maintained for a longer time to allow other cognitive processes time to function. For example, if the first word in a sentence is acoustically unclear, it may be that the semantic context provided by the following sentence will allow listeners to nevertheless correctly identify the word (Signoret et al. in press). This can only happen if a trace of the original item has been retained.
Verbal working memory is frequently measured using a reading span test (originally introduced by Daneman & Carpenter 1980) in which participants read a series of sentences and maintain a running list of the final words, which they are then asked to repeat [There are variations on the basic paradigm, such as not informing the participant whether they will be cued to recall the first or last word in a sentence (Rönnberg et al. 1989), or using memory for letters presented following each sentence rather than words from the sentence (Oswald et al. 2015). Because these differences in experimental protocol likely influence the cognitive requirements of the task, some measures may be more relevant for speech understanding than others.]. A reading span task thus requires participants to hold verbal information in mind (the list of sentence-final words) while simultaneously processing new information (the current sentence being read). Verbal working memory scores measured this way have been shown to correlate with the ability of both normal hearing and hearing-impaired listeners to process acoustically degraded speech (Lunner 2003; Rudner et al. 2011; Ward et al. 2022). Additional evidence supporting a role for verbal working memory comes from studies mentioned above in which processing degraded speech interferes with memory for previously heard words (Cousins et al. 2014; Rabbitt 1968): this result is consistent with computational models that implicate disruption of rehearsal and buffer mechanisms relied upon for memory encoding (Cousins et al. 2014; Miller & Wingfield 2010; Piquado, Cousins, et al. 2010).
Although frequently discussed as a single cognitive construct, verbal working memory has multiple components, supported through a distributed network of brain regions (Chein & Fiez 2010). Given the multiple processes supporting verbal working memory, it is important to establish whether there are behavioral or neural signatures that might allow us to more closely link specific aspects of verbal working memory with the processing of degraded speech. Obleser et al. (2012) used magnetoencephalography to compare the effects of increasing memory load during an explicit memory task and during degraded speech understanding. They found that power in the alpha band (8–13 Hz) increased with difficulty during both tasks. Importantly, there was an interaction, such that the highest memory challenge and most degraded speech the response was bigger than would be predicted from either manipulation alone. This finding is consistent with the hypothesis that both degraded speech and standard verbal working memory tasks rely on a shared, limited-capacity verbal working memory resource.
Attention-Based Performance Monitoring
An important component of completing a demanding task is monitoring our performance and adjusting our behavior to optimize success. In the context of neuroanatomically based models of attention it has been proposed that the cingulo-opercular network—comprised of the dorsal anterior cingulate and bilateral anterior insula/frontal operculum—plays an important role in this type of sustained top-down attentional control (Dosenbach et al. 2008; Eckert et al. 2009). As noted above, the cingulo-opercular network is engaged during acoustically challenging listening when participants’ performance is not perfect. Activity in the cingulo-opercular network is observed during a wide variety of tasks in which participants need to assess their performance, suggesting a domain-general role in attention-based performance monitoring (Petersen & Posner 2012). In the context of speech understanding, a performance-monitoring role for the cingulo-opercular network is also consistent with increased activity for incorrect word repetition compared with correct word repetition (Harris et al. 2009). Perhaps the most compelling neuroimaging evidence linking cingulo-opercular activity to task-relevant attentional monitoring comes from Vaden et al. (2013), who showed that cingulo-opercular activity on one trial was significantly related to performance accuracy on the following trial during word recognition in noise and subsequent memory for words (Vaden et al. 2022). In other words, the degree to which a listener engaged the cingulo-opercular network following one trial helped to predict their accuracy on the next word. Thus, although cingulo-opercular activity is unlikely necessary for all speech understanding, the cingulo-opercular network appears to play an important role in adaptive control and performance monitoring that may help listeners improve their performance during challenging listening situations (Kuchinsky et al. 2022; Vaden et al. 2015; Vaden et al. 2022).
The importance of the cingulo-opercular network during degraded speech understanding may relate to behavioral findings using the Stroop task, generally assumed to measure inhibitory control. In the classic visual Stroop task (Stroop 1935), participants must withhold an automatic response (reading a presented word) and instead name the color in which the word is written. Accuracy is typically high, but participants take longer when the written word and color are in conflict (e.g., the word “red” displayed in the blue). Functional brain imaging studies of Stroop tasks implicate the cingulo-opercular network (Leung et al. 2000; Peterson et al. 1999). Although there may be differences in the framing of attentional processes in terms of inhibition or performance monitoring, these imaging results suggest that there may be a connection between constructs of performance monitoring and inhibition as measured by the Stroop task. Individual differences in Stroop-based inhibition scores have been found in least some studies to relate to speech understanding accuracy, such that listeners with better inhibitory ability (smaller Stroop effects) perform better on speech understanding tasks than listeners with poorer inhibitory ability (Dey & Sommers 2015; Sommers & Danielson 1999; Taler et al. 2010), although this relationship depends on the specifics of the task (Knight & Heinrich 2022).
Flexible Allocation of Neurocognitive Resources
The classes of cognitive resources outlined above are theoretically and anatomically dissociable, but not mutually exclusive. That is, individual listeners may engage different processes to maximize their perception of certain kinds of speech. It is likely that the specific neurocognitive systems recruited during effortful listening are thus not static, but dynamic: The ability to flexibly allocate neurocognitive resources in an online manner allows listeners to rapidly adapt to speech processing under a wide variety of conditions.
A good example of this principle is found in the cingulo-opercular network. During successful comprehension of relatively clear speech, these regions seldom show activity above baseline levels. However, when speech is degraded to the point where a listener’s accuracy begins to decline, the cingulo-opercular network is typically engaged. Thus, the cingulo-opercular network seems to be differentially recruited when comprehension accuracy is challenged: Its involvement is transient and dependent on the acoustic clarity of the target speech, reflecting a dynamic upregulation of a discrete cognitive resource.
The principle of flexible resource allocation is illustrated in Figure 3. Speech understanding will always rely on a core network of regions involved in acoustic and lexical-semantic processing. When the acoustic clarity of the speech signal is degraded, however, additional regions need to be engaged. The resources required for understanding speech depend not only on acoustic clarity but also on the type of linguistic challenge presented by the target speech (Peelle 2012). For example, psycholinguistic factors such as word frequency or lexical competition (the number of similar-sounding words) can affect how difficult single words are to process. Understanding a spoken sentence requires semantic integration and syntactic parsing processes that are not present during single word comprehension. These are present for simple sentences but can be also be further modulated by using sentences that contain material that is grammatically complex (Peelle et al. 2010; Rodd et al. 2010; Tyler et al. 2010) or semantically ambiguous (Rodd et al. 2012). The brain networks shown in Figure 3 illustrate these different types of challenge. For example, as noted above, when speech intelligibility suffers the cingulo-opercular network is often engaged. However, when speech is acoustically degraded but still highly intelligible, premotor and prefrontal cortex is recruited. The point is that the neural and cognitive systems required to support speech understanding depend on the specific task demands (which include both linguistic and acoustic aspects).

Illustration of brain networks involved in processing clear and degraded speech. When the acoustic signal is clear (represented by the upper spectrogram), a core speech network (shaded in blue) is engaged consisting of bilateral temporal cortex and often left inferior frontal gyrus. This core speech network supports acoustic, phonological, lexico-semantic, and basic syntactic processing. When speech is degraded (represented by the lower spectrogram), the core speech network is still engaged but is complemented by additional activity required to giảm giá with the degraded speech signal. The additional regions engaged (shaded in red) depend on the specific type of cognitive support required and will likely differ as a function of the specific acoustic challenge, task demands, and cognitive and auditory ability of an individual listener.
The Role of Cognitive Factors in Explaining Individual Differences in Speech Understanding
Although the role of cognitive processing in understanding degraded speech is of interest for theoretical reasons, it also has practical implications for understanding the performance of listeners with hearing impairment, hearing aids, or cochlear implants. For example, it is well established that individual differences in speech understanding remain even after factoring out standard audiometric measures (Killion & Niquette 2000; Plomp & Mimpen 1979; Smoorenburg 1992). One possibility is that pure-tone threshold audiometric measures are insufficient to characterize hearing ability, and additional auditory measures (e.g., psychoacoustic or temporal processing) will be able to more fully explain individual differences (Humes, Busey, et al. 2013). An alternate (although not exclusive) possibility—which is my focus here—is that cognitive ability plays an important role in speech understanding. The importance of individual differences in cognitive ability is reflected by the fact that various cognitive measures (notably verbal working memory) have been found to explain significant variability in the speech understanding of listeners with and without hearing aids (Humes 2007; Humes, Kidd, et al. 2013; Lunner 2003; Rönnberg et al. 2022) and listeners with cochlear implants (Holden et al. 2013). Although hearing ability remains the strongest predictor of speech understanding accuracy, a growing number of studies affirm an important role for cognitive factors generally in explaining individual differences in speech understanding that cannot be attributed to standard audiometric differences (Akeroyd 2008). This knowledge may prove useful in guiding aural and cognitive rehabilitation (Smith et al. 2022)—for example, a listener with poor verbal working memory may benefit more from cognitive training (Richmond et al. 2011) than auditory training.
Listening Effort and Neural Plasticity
Epidemiological studies indicate that older adults with poorer hearing perform worse on cognitive tests and have an increased risk for dementia (Lin, Ferrucci, et al. 2011; Lin, Metter, et al. 2011). Although the causes for this association are still unclear, one intriguing possibility is that years of listening effort (resulting from hearing loss) may alter the brain networks engaged in speech understanding. It is unquestionably the case that our brain structure and function are affected by life experience, perhaps most evident in areas of expertise such as driving a taxi (Maguire et al. 2000) or learning new motor skills (Dayan & Cohen 2011). Given that age-related hearing loss typically develops gradually, it is reasonable to expect comparable neural reorganization might take place.
Indeed, hearing loss is associated with changes in neural processing every stage of the auditory pathway (for a review, see Peelle and Wingfield 2022). Notably, in cross-sectional studies, older adults with poorer hearing (i.e., higher pure-tone thresholds) have reduced gray matter volume in auditory cortex compared with people with better hearing (Eckert et al. 2012; Peelle et al. 2011). Less is known regarding regions outside auditory cortex. Given that executive networks such as those shown in Figure 3 are typically more active when listeners are listening to degraded speech, we might expect functional or structural changes here as well. However, it might also be the case that generally increased activity of these domain-general executive networks might have a protective effect on brain health (“use it or lose it”). Thus, although hearing loss leads to neural changes, the degree to which such plastic reorganization is related to cognitive difficulties and risk for dementia is a question that requires further investigation. This is especially true, given other plausible explanations (e.g., listeners with hearing loss may be less likely to engage in social activities, which might affect cognitive function).
CONCLUSIONS
Listening to degraded speech is a challenging task that requires listeners to devote additional cognitive resources for successful understanding, reflected in greater neural activity, increased pupil dilation, and behavior. The cognitive processes engaged when listening to acoustically degraded speech likely include verbal working memory and attention-based performance monitoring. Acoustic challenge is thus not merely an auditory problem but significantly affects a variety of cognitive operations required for both linguistic and nonlinguistic tasks. Important tasks for future studies include further clarifying which specific cognitive processes are engaged, how different types of acoustic challenge are handled by listeners, and the degree to which training or rehabilitation is able to help reduce the effects of cognitive challenge. An increased understanding of the cognitive processes required for speech understanding will help us to not only maximize speech recognition, but the productive use of this information in everyday listening.
ACKNOWLEDGMENTS
The author is grateful to Bill Clark, Lisa Davidson, Jill Firszt, Chad Rogers, and Mitch Sommers for their helpful comments on previous versions of this article.
REFERENCES
Adank PThe neural bases of difficult speech comprehension and speech production: Two Activation Likelihood Estimation (ALE) meta-analyses. Brain Lang, 2012). 122, 4254.
Akeroyd M. AAre individual differences in speech reception related to individual differences in cognitive ability? A survey of twenty experimental studies with normal and hearing-impaired adults. Int J Audiol, 2008). 47 (Suppl 2), S53S71.
Antzoulatos E. G., Miller E. KSynchronous beta rhythms of frontoparietal networks support only behaviorally relevant representations. eLife, 2022). 5, e17822.
Aston-Jones G., Cohen J. DAn integrative theory of locus coeruleus-norepinephrine function: Adaptive gain and optimal performance. Annu Rev Neurosci, 2005). 28, 403450.
Baddeley A. DWorking Memory. 1986). Oxford: Clarendon Press.
Baddeley A. DExploring the central executive. Q. J Exp Psychol, 1996). 49A, 528.
Besser J., Zekveld A. A., Kramer S. E., et al.New measures of masked text recognition in relation to speech-in-noise perception and their associations with age and cognitive abilities. J Speech Lang Hear Res, 2012). 55, 194209.
Chein J. M., Fiez J. AEvaluating models of working memory through the effects of concurrent irrelevant information. J Exp Psychol Gen, 2010). 139, 117137.
Cousins K. A., Dar H., Wingfield A., et al.Acoustic masking disrupts time-dependent mechanisms of memory encoding in word-list recall. Mem Cognit, 2014). 42, 622638.
Crinion J. T., Lambon-Ralph M. A., Warburton E. A., et al.Temporal lobe regions engaged during normal speech comprehension. Brain, 2003). 126(Pt 5), 11931201.
Daneman M., Carpenter PIndividual differences in working memory and reading. J Verbal Learning Verbal Behav, 1980). 19, 450466.
Davis M. H., Ford M. A., Kherif F., et al.Does semantic context benefit speech understanding through “top-down” processes? Evidence from time-resolved sparse fMRI. J Cogn Neurosci, 2011). 23, 39143932.
Davis M. H., Johnsrude I. SHierarchical processing in spoken language comprehension. J Neurosci, 2003). 23, 34233431.
Davis M. H., Johnsrude I. SHearing speech sounds: Top-down influences on the interface between audition and speech perception. Hear Res, 2007). 229, 132147.
Dayan E., Cohen L. GNeuroplasticity subserving motor skill learning. Neuron, 2011). 72, 443454.
DeCaro R., Peelle J. E., Grossman M., et al.The two sides of sensory-cognitive interactions: Effects of age, hearing acuity, and working memory span on sentence comprehension. Front Psychol, 2022). 7, 236.
Denes P. B., Pinson E. NThe Speech Chain: The Physics and Biology of Spoken Language. 1993). Long Grove, IL: Waveland Press, Inc.
Dey A., Sommers M. SAge-related differences in inhibitory control predict audiovisual speech perception. Psychol Aging, 2015). 30, 634646.
Dosenbach N. U., Fair D. A., Cohen A. L., et al.A dual-networks architecture of top-down control. Trends Cogn Sci, 2008). 12, 99105.
Duncan JThe multiple-demand (MD) system of the primate brain: Mental programs for intelligent behaviour. Trends Cogn Sci, 2010). 14, 172179.
Eckert M. A., Cute S. L., Vaden K. I. Jr, et al.Auditory cortex signs of age-related hearing loss. J Assoc Res Otolaryngol, 2012). 13, 703713.
Eckert M. A., Menon V., Walczak A., et al.At the heart of the ventral attention system: The right anterior insula. Hum Brain Mapp, 2009). 30, 25302541.
Eckert M. A., Teubner-Rhodes S., Vaden K. I. JrIs listening in noise worth it? The neurobiology of speech recognition in challenging listening conditions. Ear Hear, 2022). 37(Suppl 1), 101S110S.
Erb J., Henry M. J., Eisner F., et al.The brain dynamics of rapid perceptual adaptation to adverse listening conditions. J Neurosci, 2013). 33, 1068810697.
Evans S., Kyong J. S., Rosen S., et al.The pathways for intelligible speech: multivariate and univariate perspectives. Cereb Cortex, 2014). 24, 23502361.
Evans S., McGettigan CComprehending auditory speech: Previous and potential contributions of functional MRI. Lang Cogn Neurosci, 2022). 32, 829846.
Evans S., McGettigan C., Agnew Z. K., et al.Getting the cocktail party started: Masking effects in speech perception. J Cogn Neurosci, 2022). 28, 483500.
Gatehouse S., Gordon JResponse times to speech stimuli as measures of benefit from amplification. Br J Audiol, 1990). 24, 6368.
Grady CThe cognitive neuroscience of ageing. Nat Rev Neurosci, 2012). 13, 491505.
Harris K. C., Dubno J. R., Keren N. I., et al.Speech recognition in younger and older adults: A dependency on low-level auditory cortex. J Neurosci, 2009). 29, 60786087.
Hassanpour M. S., Eggebrecht A. T., Culver J. P., et al.Mapping cortical responses to speech using high-density diffuse optical tomography. Neuroimage, 2015). 117, 319326.
Heinrich A., Schneider B. AElucidating the effects of ageing on remembering perceptually distorted word pairs. Q. J Exp Psychol (Hove), 2011). 64, 186205.
Heinrich A., Schneider B. A., Craik F. IInvestigating the influence of continuous babble on auditory short-term memory performance. Q. J Exp Psychol (Hove), 2008). 61, 735751.
Holden L. K., Finley C. C., Firszt J. B., et al.Factors affecting open-set word recognition in adults with cochlear implants. Ear Hear, 2013). 34, 342360.
Hornsby B. W., Naylor G., Bess F. HA taxonomy of fatigue concepts and their relation to hearing loss. Ear Hear, 2022). 37(Suppl 1), 136S144S.
Humes L. EThe contributions of audibility and cognitive factors to the benefit provided by amplified speech to older adults. J Am Acad Audiol, 2007). 18, 590603.
Humes L. E., Busey T. A., Craig J., et al.Are age-related changes in cognitive function driven by age-related changes in sensory processing? Atten Percept Psychophys, 2013). 75, 508524.
Humes L. E., Kidd G. R., Lentz J. JAuditory and cognitive factors underlying individual differences in aided speech-understanding among older adults. Front Syst Neurosci, 2013). 7, 55.
Kahneman D., Beatty JPupil diameter and load on memory. Science, 1966). 154, 15831585.
Killion M. C., Niquette P. AWhat can the pure-tone audiogram tell us about a patient’s SNR loss? Hearing J, 2000). 53, 4653.
Knight S., Heinrich ADifferent measures of auditory and visual stroop interference and their relationship to speech intelligibility in noise. Front Psychol, 2022). 8, 230.
Koss M. CPupillary dilation as an index of central nervous system alpha 2-adrenoceptor activation. J Pharmacol Methods, 1986). 15, 119.
Kramer S. E., Kapteyn T. S., Festen J. M., et al.Assessing aspects of auditory handicap by means of pupil dilatation. Audiology, 1997). 36, 155164.
Kramer S. E., Zekveld A. A., Houtgast TMeasuring cognitive factors in speech comprehension: the value of using the Text Reception Threshold test as a visual equivalent of the SRT test. Scand J Psychol, 2009). 50, 507515.
Kuchinsky S. E., Ahlstrom J. B., Vaden K. I. Jr, et al.Pupil size varies with word listening and response selection difficulty in older adults with hearing loss. Psychophysiology, 2013). 50, 2334.
Kuchinsky S. E., Vaden K. I. Jr, Ahlstrom J. B., et al.Task-related vigilance during word recognition in noise for older adults with hearing loss. Exp Aging Res, 2022). 42, 5066.
Kukla AFoundations of an attributional theory of performance. Psychological Review, 1972). 79, 454470.
Laeng B., Sirois S., Gredebäck GPupillometry: A window to the preconscious? Perspect Psychol Sci, 2012). 7, 1827.
Lee Y. S., Min N. E., Wingfield A., et al.Acoustic richness modulates the neural networks supporting intelligible speech processing. Hear Res, 2022). 333, 108117.
Leung H. C., Skudlarski P., Gatenby J. C., et al.An sự kiện-related functional MRI study of the stroop color word interference task. Cereb Cortex, 2000). 10, 552560.
Lin F. R., Ferrucci L., Metter E. J., et al.Hearing loss and cognition in the Baltimore Longitudinal Study of Aging. Neuropsychology, 2011). 25, 763770.
Lin F. R., Metter E. J., O’Brien R. J., et al.Hearing loss and incident dementia. Arch Neurol, 2011). 68, 214220.
Luce P. A., Pisoni D. BRecognizing spoken words: The neighborhood activation model. Ear Hear, 1998). 19, 136.
Lunner TCognitive function in relation to hearing aid use. Int J Audiol, 2003). 42(Suppl 1), S49S58.
Maguire E. A., Gadian D. G., Johnsrude I. S., et al.Navigation-related structural change in the hippocampi of taxi drivers. Proc Natl Acad Sci, 2000). 97, 43984403.
Marslen-Wilson W., Tyler L. KThe temporal structure of spoken language understanding. Cognition, 1980). 8, 171.
Mattys S. L., Davis M. H., Bradlow A. R., et al.Speech recognition in adverse conditions: A review. Lang Cogn Process, 2012). 27, 953978.
McCoy S. L., Tun P. A., Cox L. C., et al.Hearing loss and perceptual effort: Downstream effects on older adults’ memory for speech. Q. J Exp Psychol A, 2005). 58, 2233.
McGettigan C., Evans S., Rosen S., et al.An application of univariate and multivariate approaches in FMRI to quantifying the hemispheric lateralization of acoustic and linguistic processes. J Cogn Neurosci, 2012). 24, 636652.
Miller P., Wingfield ADistinct effects of perceptual quality on auditory word recognition, memory formation and recall in a neural model of sequential memory. Front Syst Neurosci, 2010). 4, 14.
Mirman DGrowth Curve Analysis and Visualization Using R. 2014). Tp New York, NY: Chapman & Hall/CRC.
Murphy D. R., Craik F. I. M., Li K. Z. H., et al.Comparing the effects of aging and background noise on short-term memory performance. Psychol Aging, 2000). 15, 323334.
Neta M., Miezin F. M., Nelson S. M., et al.Spatial and temporal characteristics of error-related activity in the human brain. J Neurosci, 2015). 35, 253266.
Obleser J., Wise R. J., Dresner M. A., et al.Functional integration across brain regions improves speech perception under adverse listening conditions. J Neurosci, 2007). 27, 22832289.
Obleser J., Wöstmann M., Hellbernd N., et al.Adverse listening conditions and memory load drive a common α oscillatory network. J Neurosci, 2012). 32, 1237612383.
Okada K., Rong F., Venezia J., et al.Hierarchical organization of human auditory cortex: Evidence from acoustic invariance in the response to intelligible speech. Cereb Cortex, 2010). 20, 24862495.
Oswald F. L., McAbee S. T., Redick T. S., et al.The development of a short domain-general measure of working memory capacity. Behav Res Methods, 2015). 47, 13431355.
Peelle J. EThe hemispheric lateralization of speech processing depends on what “speech” is: A hierarchical perspective. Front Hum Neurosci, 2012). 6, 309.
Peelle J. EMethodological challenges and solutions in auditory functional magnetic resonance imaging. Front Neurosci, 2014). 8, 253.
Peelle J. EOptical neuroimaging of spoken language. Lang Cogn Neurosci, 2022). 32, 847854.
Peelle J. E., Eason R. J., Schmitter S., et al.Evaluating an acoustically quiet EPI sequence for use in fMRI studies of speech and auditory processing. Neuroimage, 2010). 52, 14101419.
Peelle J. E., Johnsrude I. S., Davis M. HHierarchical processing for speech in human auditory cortex and beyond. Front Hum Neurosci, 2010). 4, 51.
Peelle J. E., Troiani V., Grossman M., et al.Hearing loss in older adults affects neural systems supporting speech comprehension. J Neurosci, 2011). 31, 1263812643.
Peelle J. E., Troiani V., Wingfield A., et al.Neural processing during older adults’ comprehension of spoken sentences: Age differences in resource allocation and connectivity. Cereb Cortex, 2010). 20, 773782.
Peelle J. E., Wingfield AThe neural consequences of age-related hearing loss. Trends Neurosci, 2022). 39, 486497.
Petersen S. E., Posner M. IThe attention system of the human brain: 20 years after. Annu Rev Neurosci, 2012). 35, 7389.
Peterson B. S., Skudlarski P., Gatenby J. C., et al.An fMRI study of Stroop word-color interference: Evidence for cingulate subregions subserving multiple distributed attentional systems. Biol Psychiatry, 1999). 45, 12371258.
Pichora-Fuller M. KHow social psychological factors may modulate auditory and cognitive functioning during listening. Ear Hear, 2022). 37(Suppl 1), 92S100S.
Pichora-Fuller M. K., Kramer S. E., Eckert M. A., et al.Hearing impairment and cognitive energy: The framework for understanding effortful listening (FUEL). Ear Hear, 2022). 37, 5S27S.
Pichora-Fuller M. K., Schneider B. A., Daneman MHow young and old adults listen to and remember speech in noise. J Acoust Soc Am, 1995). 97, 593608.
Piquado T., Benichov J. I., Brownell H., et al.The hidden effect of hearing acuity on speech recall, and compensatory effects of self-paced listening. Int J Audiol, 2012). 51, 576583.
Piquado T., Cousins K. A., Wingfield A., et al.Effects of degraded sensory input on memory for speech: Behavioral data and a test of biologically constrained computational models. Brain Res, 2010). 1365, 4865.
Piquado T., Isaacowitz D., Wingfield APupillometry as a measure of cognitive effort in younger and older adults. Psychophysiology, 2010). 47, 560569.
Plomp R., Mimpen A. MSpeech-reception threshold for sentences as a function of age and noise level. J Acoust Soc Am, 1979). 66, 13331342.
Power J. D., Petersen S. EControl-related systems in the human brain. Curr Opin Neurobiol, 2013). 23, 223228.
Rabbitt P. MChannel-capacity, intelligibility and immediate memory. Q. J Exp Psychol, 1968). 20, 241248.
Rabbitt P. M. AMild hearing loss can cause apparent memory failures which increase with age and reduce with IQ. Acta Otolaryngolica, 1991). 476, 167176.
Richmond L. L., Morrison A. B., Chein J. M., et al.Working memory training and transfer in older adults. Psychol Aging, 2011). 26, 813822.
Richter MThe moderating effect of success importance on the relationship between listening demand and listening effort. Ear Hear, 2022). 37(Suppl 1), 111S117S.
Rodd J. M., Davis M. H., Johnsrude I. SThe neural mechanisms of speech comprehension: fMRI studies of semantic ambiguity. Cereb Cortex, 2005). 15, 12611269.
Rodd J. M., Johnsrude I. S., Davis M. HDissociating frontotemporal contributions to semantic ambiguity resolution in spoken sentences. Cereb Cortex, 2012). 22, 17611773.
Rodd J. M., Longe O. A., Randall B., et al.The functional organisation of the fronto-temporal language system: Evidence from syntactic and semantic ambiguity. Neuropsychologia, 2010). 48, 13241335.
Rönnberg J., Arlinger S., Lyxell B., et al.Visual evoked potentials: Relation to adult speechreading and cognitive function. J Speech Hear Res, 1989). 32, 725735.
Rönnberg J., Lunner T., Ng E. H., et al.Hearing impairment, cognition and speech understanding: Exploratory factor analyses of a comprehensive test battery for a group of hearing aid users, the n200 study. Int J Audiol, 2022). 55, 623642.
Rönnberg J., Lunner T., Zekveld A., et al.The ease of language understanding (ELU) model: theoretical, empirical, and clinical advances. Front Syst Neurosci, 2013). 7, 31.
Rönnberg J., Rudner M., Foo C., et al.Cognition counts: A working memory system for ease of language understanding (ELU). Int J Audiol, 2008). 47(Suppl 2), S99105.
Rudner M., Rönnberg J., Lunner TWorking memory supports listening in noise for persons with hearing impairment. J Am Acad Audiol, 2011). 22, 156167.
Scott S. K., Blank C. C., Rosen S., et al.Identification of a pathway for intelligible speech in the left temporal lobe. Brain, 2000). 123(Pt 12), 24002406.
Shannon R. V., Zeng F. G., Kamath V., et al.Speech recognition with primarily temporal cues. Science, 1995). 270, 303304.
Signoret C., Johnsrude I., Classon E, et al.Combined effects of form- and meaning-based predictability on perceived clarity of speech. J Exp Psychol Hum Percept Perform.In press).
Smith S. L., Pichora-Fuller M. K., Alexander GDevelopment of the word auditory recognition and recall measure: A working memory test for use in rehabilitative audiology. Ear Hear, 2022). 37, e360e376.
Smoorenburg G. FSpeech reception in quiet and in noisy conditions by individuals with noise-induced hearing loss in relation to their tone audiogram. J Acoust Soc Am, 1992). 91, 421437.
Sommers M. SThe structural organization of the mental lexicon and its contribution to age-related declines in spoken-word recognition. Psychol Aging, 1996). 11, 333341.
Sommers M. S., Danielson S. MInhibitory processes and spoken word recognition in young and older adults: The interaction of lexical competition and semantic context. Psychol Aging, 1999). 14, 458472.
Stokes M. G., Kusunoki M., Sigala N., et al.Dynamic coding for cognitive control in prefrontal cortex. Neuron, 2013). 78, 364375.
Stroop JStudies of interference in serial verbal reactions. J Exp Psychol, 1935). 18, 643662.
Surprenant A. MThe effect of noise on memory for spoken syllables. Int J Psychol, 1999). 34, 328333.
Taler V., Aaron G. P., Steinmetz L. G., et al.Lexical neighborhood density effects on spoken word recognition and production in healthy aging. J Gerontol B Psychol Sci Soc Sci, 2010). 65, 551560.
Tyler L. K., Shafto M. A., Randall B., et al.Preserving syntactic processing across the adult life span: The modulation of the frontotemporal language system in the context of age-related atrophy. Cereb Cortex, 2010). 20, 352364.
Vaden K. I. Jr, Kuchinsky S. E., Ahlstrom J. B., et al.Cortical activity predicts which older adults recognize speech in noise and when. J Neurosci, 2015). 35, 39293937.
Vaden K. I. Jr, Kuchinsky S. E., Ahlstrom J. B., et al.Cingulo-opercular function during word recognition in noise for older adults with hearing loss. Exp Aging Res, 2022). 42, 6782.
Vaden K. I. Jr, Kuchinsky S. E., Cute S. L., et al.The cingulo-opercular network provides word-recognition benefit. J Neurosci, 2013). 33, 1897918986.
Vaden K. I. Jr, Teubner-Rhodes S., Ahlstrom J. B., et al.Cingulo-opercular activity affects incidental memory encoding for speech in noise. Neuroimage, 2022). 157, 381387.
Van Engen K. J., Peelle J. EListening effort and accented speech. Front Hum Neurosci, 2014). 8, 577.
Ward C. M., Rogers C. S., Van Engen K. J., et al.Effects of age, acoustic challenge, and verbal working memory on recall of narrative speech. Exp Aging Res, 2022). 42, 126144.
Wild C. J., Davis M. H., Johnsrude I. SHuman auditory cortex is sensitive to the perceived clarity of speech. Neuroimage, 2012). 60, 14901502.
Wild C. J., Yusuf A., Wilson D. E., et al.Effortful listening: The processing of degraded speech depends critically on attention. J Neurosci, 2012). 32, 1401014021.
Wingfield AEvolution of models of working memory and cognitive resources. Ear Hear, 2022). 37(Suppl 1), 35S43S.
Wingfield A., Amichetti N. M., Lash ACognitive aging and hearing acuity: Modeling spoken language comprehension. Front Psychol, 2015). 6, 684.
Wingfield A., Grossman MLanguage and the aging brain: Patterns of neural compensation revealed by functional brain imaging. J Neurophysiol, 2006). 96, 28302839.
Wingfield A., McCoy S. L., Peelle J. E., et al.Effects of adult aging and hearing loss on comprehension of rapid speech varying in syntactic complexity. J Am Acad Audiol, 2006). 17, 487497.
Wingfield A., Tun P. A., Koh C. K., et al.Regaining lost time: Adult aging and the effect of time restoration on recall of time-compressed speech. Psychol Aging, 1999). 14, 380389.
Wingfield A., Tun P. A., McCoy S. LHearing loss in older adulthood: What it is and how it interacts with cognitive performance. Curr Dir Psychol Sci, 2005). 14, 144148.
Wöstmann M., Fiedler L., Obleser JTracking the signal, cracking the code: Speech and speech comprehension in non-invasive human electrophysiology. Lang Cogn Neurosci, 2022). 32, 855869.
Zekveld A. A., George E. L., Kramer S. E., et al.The development of the text reception threshold test: A visual analogue of the speech reception threshold test. J Speech Lang Hear Res, 2007). 50, 576584.
Zekveld A. A., Kramer S. ECognitive processing load across a wide range of listening conditions: Insights from pupillometry. Psychophysiology, 2014). 51, 277284.
Zekveld A. A., Kramer S. E., Festen J. MPupil response as an indication of effortful listening: The influence of sentence intelligibility. Ear Hear, 2010). 31, 480490.
Zekveld A. A., Kramer S. E., Festen J. MCognitive load during speech perception in noise: The influence of age, hearing loss, and cognition on the pupil response. Ear Hear, 2011). 32, 498510.
Keywords:
Acoustic challenge; Aging; Listening effort; Speech comprehension; Working memory
Copyright © 2022 The Authors. Ear & Hearing is published on behalf of the American Auditory Society, by Wolters Kluwer Health, Inc.
Post a Comment